In today’s rapidly evolving digital landscape, generative AI has emerged as a transformative force, reshaping how businesses operate and create value. This cutting-edge technology enables machines to produce AI-generated content—from text and images to music and videos—at an unprecedented scale and speed. Powered by generative AI models, deep learning, and AI technologies, it allows businesses to automate complex tasks, enhance creativity, and optimize workflows in real-time.
For entrepreneurs, market leaders, and CEOs, generative AI offers a competitive edge by streamlining content creation, improving customer engagement, and driving innovation. However, as adoption grows, so do concerns about security risks and data-related challenges.
This blog explores the far-reaching impact of generative AI, focusing on three critical aspects: its effect on security, its primary advantage in content creation, and the data challenges it faces. Whether you're scaling a startup or leading an enterprise, understanding generative AI’s potential is key to staying ahead in today’s tech-driven world.
What is Generative AI and How Does It Work?
Generative AI refers to a class of artificial intelligence that creates new content by learning patterns from vast datasets. Unlike traditional AI, which primarily analyzes and processes existing information, a generative model can generate entirely new text, images, music, and even code.
At the heart of generative AI are neural networks, specifically generative adversarial networks (GANs) and large language models (LLMs). GANs consist of two competing networks—a generator that creates data and a discriminator that evaluates its authenticity—enabling AI to produce highly realistic outputs. Meanwhile, LLMs like GPT-4 are trained on massive amounts of training data to understand language patterns and generate human-like text.
Popular generative AI tools include:
- Image Generators: Tools like DALL·E and MidJourney create high-quality images from text prompts.
- Text-Based Models: ChatGPT and Gemini generate articles, summaries, and even creative writing.
- Code Generators: GitHub Copilot assists developers by predicting and completing code snippets.
By leveraging LLMs and vast training data, generative AI is revolutionizing industries, offering automation and creativity at an unprecedented scale. However, while its applications are vast, businesses must also consider the ethical and security challenges that come with it.
Real-World Applications and Benefits of Generative AI Across Industries
Generative AI is revolutionizing industries by automating processes, enhancing creativity, and delivering real-time insights. From startups to large enterprises, AI-powered tools are driving innovation, reducing costs, and improving efficiency.
Entrepreneurs and Startups
For business leaders and startup founders, generative AI tools offer a competitive edge by streamlining operations and boosting engagement.
- Automating customer service: AI chatbots powered by neural networks handle repetitive inquiries, improving response times and allowing teams to focus on strategic growth.
- Personalized marketing and social media posts: AI-generated content helps startups create dynamic, audience-specific campaigns across platforms.
- Product development acceleration: AI-driven prototyping reduces time-to-market by rapidly generating design variations and predicting market responses.
SaaS Leaders
For SaaS businesses, AI enhances user experience and marketing efficiency.
- Improved UI/UX design: AI tools suggest design optimizations, analyze user interactions, and enhance usability.
- Real-time analytics: AI-powered insights help SaaS companies refine products and optimize customer experiences.
- Scalable content creation: AI streamlines writing blogs, whitepapers, and case studies, strengthening brand authority and customer education.
Crypto and Web3 Builders
Blockchain and Web3 projects benefit significantly from AI-generated content and automation.
- NFT creation: Image generators assist in designing unique NFT artwork.
- Community engagement: AI-driven content boosts engagement on Discord, Twitter, and other social platforms.
- Automating tokenomics analysis: AI models analyze token performance, generating reports for investors and stakeholders.
Healthcare
Generative AI is transforming healthcare by improving efficiency and personalizing treatments.
- Accelerating drug discovery: AI analyzes massive datasets to identify potential drug candidates faster.
- Personalized treatment plans: AI evaluates patient history to recommend customized treatments and medication.
- Medical simulations: AI-generated medical models help doctors practice complex surgeries and diagnose rare conditions.
Creative Industries
Generative AI enhances content creation for artists, musicians, and filmmakers.
- Music and video production: AI tools generate background scores, special effects, and automated video edits.
- AI-generated artwork: Image generators assist in designing posters, branding, and digital art.
- Screenwriting and storytelling: AI tools help writers develop scripts, character arcs, and plotlines.
Manufacturing
Generative AI is streamlining production processes and predictive maintenance.
- Product design optimization: AI simulates different design iterations to enhance functionality and durability.
- Predictive maintenance: AI detects equipment failures before they happen, minimizing downtime.
- Supply chain efficiency: AI forecasts demand and optimizes inventory management.
Education
AI is enhancing learning experiences by making education more interactive and accessible.
- Personalized learning materials: AI tailors lesson plans to students' learning speeds and preferences.
- Automated grading: AI speeds up the evaluation process, allowing teachers to focus on instruction.
- Interactive learning tools: AI generates quizzes, simulations, and virtual tutoring systems.
Finance
Generative AI is enhancing financial decision-making and security measures.
- Fraud detection: AI analyzes transaction patterns to identify fraudulent activities in real time.
- Automated financial reports: AI generates reports for audits, forecasting, and budgeting.
- Optimized investment strategies: AI evaluates market trends to recommend profitable investment opportunities.
Retail
AI is redefining the shopping experience and enhancing operational efficiency.
- Personalized recommendations: AI suggests products based on consumer behavior and preferences.
- Automated product descriptions: AI-generated descriptions optimize SEO and improve customer engagement.
- Inventory management: AI predicts demand and adjusts stock levels accordingly.
Gaming
Generative AI is revolutionizing game development and player engagement.
- Character and environment design: AI creates realistic characters and immersive gaming worlds.
- Dynamic storylines: AI generates evolving narratives based on player choices.
- AI-powered NPCs: Non-player characters (NPCs) react more intelligently, improving gameplay realism.
Legal
AI is streamlining legal processes and improving document management.
- Contract drafting: AI generates standard legal agreements with minimal human input.
- Case law analysis: AI scans legal databases for relevant precedents, speeding up research.
- Document review automation: AI simplifies contract analysis, reducing errors and manual workload.
Agriculture
AI is enhancing farming efficiency and sustainability.
- Crop yield prediction: AI analyzes weather and soil conditions to forecast harvests.
- Automated farm management: AI-powered tools optimize irrigation, fertilization, and pest control.
- Weather pattern analysis: AI predicts climate changes to help farmers plan accordingly.
Across industries, generative AI technologies are driving innovation, reducing operational costs, and improving efficiency, making businesses more competitive in the modern world.
The Primary Advantage of Using Generative AI in Content Creation
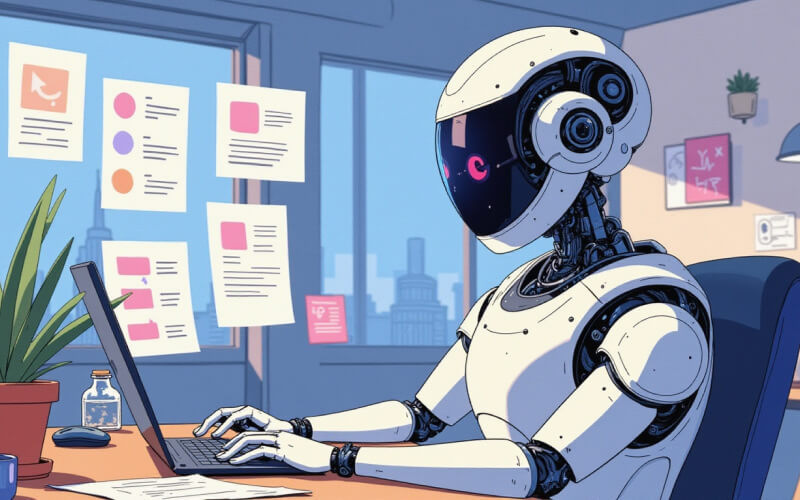
Generative AI is revolutionizing AI for content creation by enabling businesses to produce high-quality AI-generated content at scale. Whether it’s blog articles, social media posts, videos, or marketing copy, AI-powered tools are enhancing branding, engagement, and efficiency in real-time.
Diverse Types of Content at Scale
Generative AI can create a variety of content formats tailored for different platforms:
- Blogs and articles: AI tools like GPT-based models generate long-form, SEO-optimized articles quickly.
- Social media posts: AI assists in crafting engaging captions, tweets, and posts that align with a brand’s voice.
- Videos and visuals: AI-powered tools automate video scripts, subtitles, and even video creation itself.
The Primary Advantage: Cost-Efficiency, Scalability, and Creativity
For startups and SaaS companies with limited resources, AI-driven content creation is a game-changer:
- Cost-Efficiency: Reduces reliance on expensive content teams while maintaining quality.
- Scalability: AI can generate hundreds of posts, emails, and ads in seconds.
- Enhanced Creativity: AI models suggest unique ideas, helping brands stand out in competitive markets
Examples of AI-Generated Content in Branding
- Startups use AI to create compelling product descriptions and website copy.
- SaaS businesses automate blog generation to educate users and attract leads.
- E-commerce brands leverage AI to craft personalized marketing emails and ad copy.
By leveraging AI technologies, businesses can maintain a consistent content strategy while saving time and resources—driving engagement and growth in an increasingly competitive digital landscape.
How Has Generative AI Affected Security?
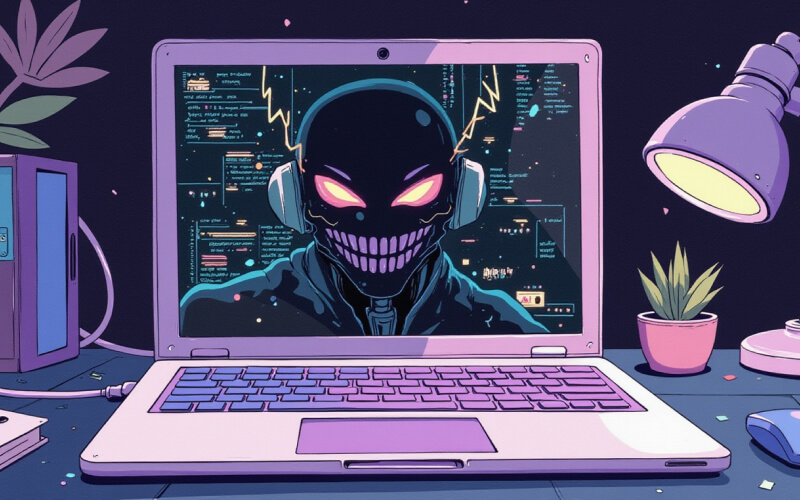
Generative AI is reshaping the security landscape, playing a dual role as both a powerful cybersecurity tool and a potential risk. Its advanced capabilities can enhance threat detection and simulate attacks for better defense strategies, but at the same time, bad actors can misuse AI tools for malicious activities.
Generative AI as a Security Asset
AI-powered generative models are being used to strengthen cybersecurity by:
- Identifying vulnerabilities: AI can scan systems for weak points, predicting where attacks might occur.
- Simulating cyberattacks: Ethical hackers use AI to create real-world cyberattack scenarios to test security defenses.
- Enhancing threat detection: Neural network-based AI tools analyze patterns to detect anomalies and prevent data breaches.
Security Threats Posed by Generative AI
While AI improves security, malicious actors exploit it for cybercrime:
- Deepfakes: AI-generated fake videos and images are used for misinformation and fraud.
- AI-powered phishing: Hackers use AI to craft realistic emails and messages to deceive users.
- Automated hacking: AI can generate malware capable of bypassing security measures.
Mitigating Security Risks for Entrepreneurs & Crypto Builders
To protect businesses and digital assets, proactive measures include:
- AI-driven monitoring: Implementing AI-powered security tools to detect and neutralize threats in real-time.
- Multi-layered authentication: Strengthening identity verification to prevent unauthorized access.
- Regulatory compliance: Following security best practices and ensuring AI tools align with ethical guidelines.
By leveraging AI responsibly, businesses can harness its power for security while mitigating its risks—ensuring a safer digital environment.
What Challenge Does Generative AI Face with Respect to Data?
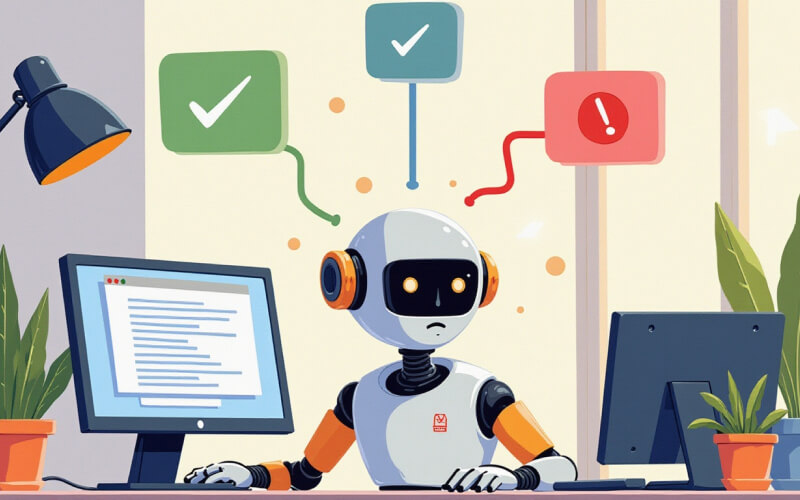
Generative AI's power hinges on the quality and availability of training data, yet several challenges can hinder its effectiveness. These challenges include ensuring data diversity, managing biases, and safeguarding privacy. Additionally, fine-tuning AI models is essential to maintain accuracy and relevance, but this process can be complex and resource-intensive.
Ensuring Data Quality and Diversity
Reliable AI requires high-quality and diverse datasets. Without this, generative AI can produce outputs that are either inaccurate or limited in scope. It's crucial to ensure that data sets are representative of various perspectives to avoid narrow and potentially flawed conclusions.
Addressing AI Bias
Generative AI models often inherit biases present in their training data. If the data reflects past inequities or imbalances, AI systems can inadvertently perpetuate these issues. Startups and SaaS companies must actively work to address these hidden biases and avoid reinforcing harmful stereotypes.
Balancing Privacy and Innovation
With growing concerns around data privacy, businesses must ensure that AI models are not infringing on user trust. Striking a balance between leveraging data for AI innovation and respecting privacy is essential. This is especially true in industries like crypto and Web3, where data security is paramount.
Updating AI for Relevance
AI models must evolve with the times. Regular fine-tuning and updates are critical to keep AI tools relevant. This ensures that generative AI remains adaptable to the real world and responsive to new data, changes, and user feedback.
Strengthening Data Governance
Solutions like leveraging diverse datasets and putting in place data governance frameworks help ensure AI is ethically sound and compliant with laws. By involving communities in the development process, businesses can design more inclusive, transparent, and effective AI systems.
By addressing these challenges, businesses can maximize the potential of generative AI while fostering trust and delivering meaningful, real-time results.
Conclusion
Generative AI has the potential to revolutionize industries, offering significant benefits like enhanced content creation, real-time solutions, and improved business efficiency. By leveraging AI-generated content and large language models (LLMs), businesses can scale quickly, automate tasks, and enhance customer experiences. However, it’s crucial to recognize the challenges, such as ensuring data quality, addressing biases, and managing security risks. Entrepreneurs, investors, and innovators should embrace generative AI as a powerful tool for growth, while remaining mindful of its limitations and ethical implications. By strategically implementing generative AI, companies can gain a competitive edge, drive innovation, and stay ahead in an ever-evolving market.
Frequently Asked Questions
The primary feature of Generative AI is its ability to create new and unique content based on patterns learned from massive datasets. Unlike traditional AI models that analyze and classify data, generative AI produces text, images, music, code, and even videos in real time. This capability is powered by advanced neural networks, such as Generative Adversarial Networks (GANs) and Large Language Models (LLMs), which enable AI to understand and mimic human-like creativity.
Generative AI enhances automation, personalization, and efficiency across various industries. For example, businesses use it to generate marketing content, automate customer support with AI chatbots, and even create synthetic data for research. Its ability to adapt and produce contextually relevant outputs makes it invaluable for streamlining workflows, improving productivity, and driving innovation. However, while it offers immense potential, challenges like data bias and ethical concerns remain key considerations.
The key difference between Generative AI and Predictive AI lies in their purpose and output.
- Generative AI creates new content by learning patterns from large datasets. It produces text, images, music, and code, enabling applications like AI-generated art, chatbots, and content automation. Examples include ChatGPT, DALL·E, and MidJourney.
- Predictive AI analyzes historical data to make forecasts and recommendations. It identifies trends, detects anomalies, and optimizes decision-making in areas like finance, healthcare, and marketing. Examples include fraud detection models, recommendation systems, and demand forecasting tools.
While Predictive AI focuses on “what will happen next”, Generative AI focuses on “creating something new.” Both play crucial roles in modern AI applications, often working together to enhance automation and efficiency.
Copyright law around AI-generated images is still evolving, but key concerns include ownership, originality, and fair use.
- Ownership – Traditional copyright laws require human authorship. Since AI lacks legal personhood, most jurisdictions do not grant copyright to fully AI-generated works. However, human involvement in prompting and refining the image may influence ownership claims.
- Originality – AI-generated content is trained on existing works, raising concerns about potential copyright infringement if it replicates or closely resembles copyrighted material.
- Fair Use & Licensing – Using AI-generated images commercially may require checking for licensing terms or ensuring they do not directly copy protected content. Some platforms restrict AI-generated works from being copyrighted altogether.
As laws adapt, businesses and creators should stay updated on AI-related intellectual property policies to avoid legal risks.
Generative AI is transforming drug discovery by accelerating research, reducing costs, and improving accuracy. Key roles include:
- Molecular Design & Drug Discovery – AI models generate novel molecular structures with potential therapeutic properties, speeding up the identification of new drug candidates.
- Protein Structure Prediction – Tools like AlphaFold predict protein folding, helping researchers understand disease mechanisms and design targeted treatments.
- Drug Repurposing – AI analyzes existing drugs to identify new uses, reducing development time and costs.
- Clinical Trial Optimization – AI models predict patient responses, improving trial design and success rates.
By leveraging AI-driven simulations and large-scale data analysis, pharmaceutical companies can innovate faster, improving healthcare outcomes.
Generative AI raises several ethical concerns, including:
- Bias and Fairness – AI models can inherit biases from training data, leading to unfair or discriminatory outcomes. Ensuring diverse datasets and ethical AI practices is crucial.
- Misinformation and Deepfakes – AI-generated content can spread false information, impacting trust in media, politics, and security. Regulation and watermarking AI content help mitigate risks.
- Intellectual Property & Copyright – AI-generated content raises legal questions about ownership and attribution, requiring updated copyright laws and ethical guidelines.
- Privacy & Data Security – AI models rely on vast amounts of data, often including sensitive information, making privacy protection and compliance with regulations like GDPR essential.
- Job Displacement – Automation may replace human roles, requiring workforce adaptation, reskilling, and responsible AI deployment.
Balancing innovation with ethical responsibility ensures AI benefits society while minimizing harm.
Prompt engineering is crucial for optimizing the performance of generative AI models. It involves designing precise and structured inputs (prompts) to guide AI in producing accurate, relevant, and high-quality outputs.
- Improves Output Quality – Well-crafted prompts help generate more coherent, contextually relevant, and refined responses.
- Enhances Control & Customization – By adjusting wording, tone, and structure, users can influence AI-generated content to meet specific needs.
- Reduces Errors & Bias – Strategic prompts help minimize hallucinations (false information) and biases in AI responses.
- Boosts Efficiency – Effective prompts reduce the need for multiple iterations, saving time and resources in content creation, coding, and automation.
- Expands AI Applications – Prompt engineering enables AI to perform diverse tasks, from generating text and images to answering complex queries and automating workflows.
Mastering prompt engineering is key to unlocking the full potential of generative AI.